
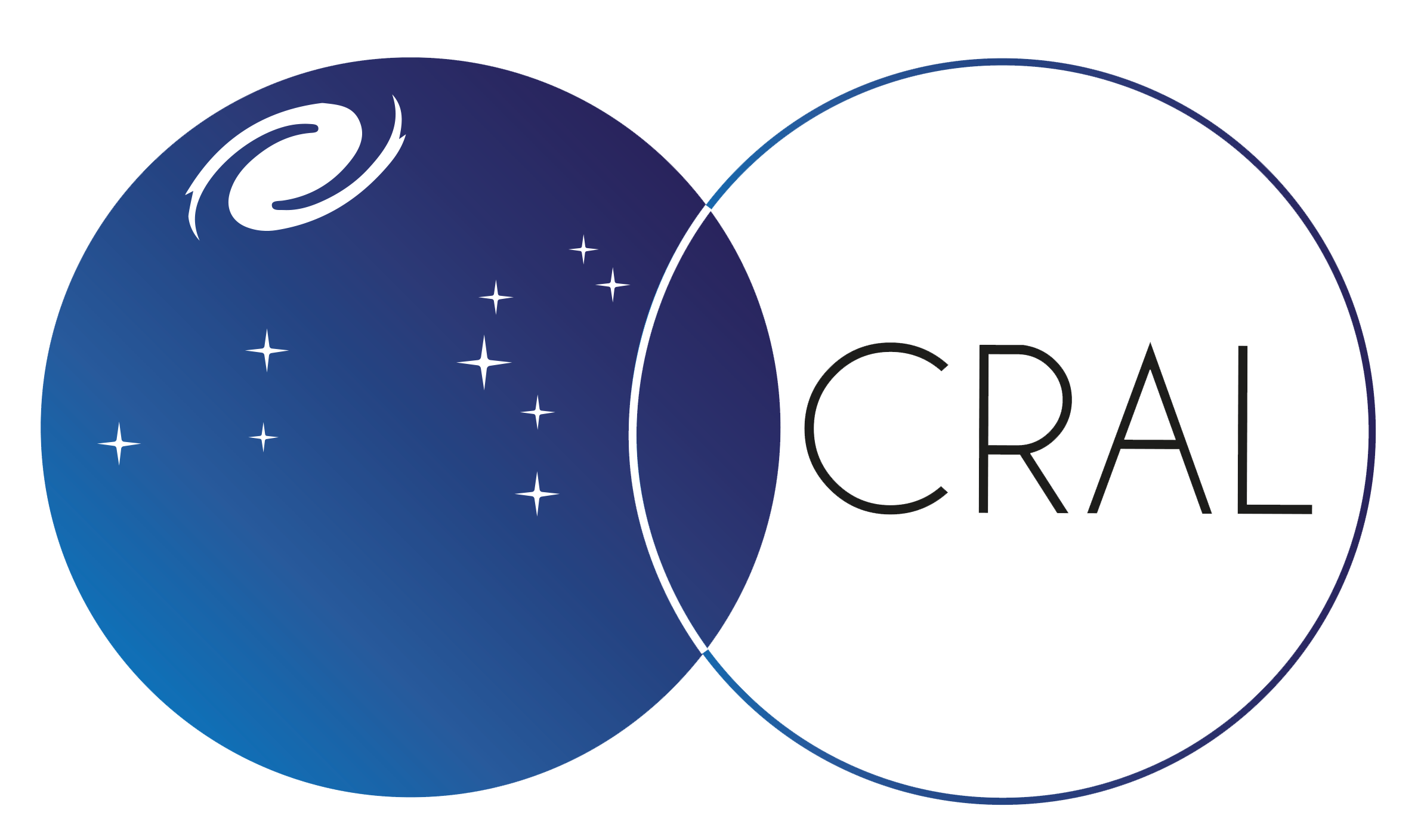
CRAL@Obs PhD Defense by Théo Santos (CRAL, LGL)
Generative neural networks for parametrization in inverse methods: applications in astrophysics and geophysics
In physical science, it is common to study the characteristics of an object that are not directly available but which certain effects within the physical system are observable. The observations can then be used to deduce the properties of the object of interest: the approach known as “inverse” focuses on such problems. An essential question is the parametrization: how to properly define the parameters that define the object to study? The choice is crucial, as it determines the formulation of the inverse problem and therefore affects its difficulty to solve.
In recent years, machine learning, particularly generative neural networks, has offered a promising opportunity for addressing parametrization. These generative networks can generate, at will, objects similar to training objects, from only a few input parameters. A generative network can thus be trained on a set of possible objects and then used for parametrization. This approach presents several advantages: it is computationally efficient, it attenuates the irregularities between the parameters defining the object and the observations, it constrains the problem to a class of models similar to those in the training set, and it requires few degrees of freedom.
This thesis is devoted to the methodological analysis of this parametrization. In particular, I propose various tools to ensure the optimal training of a generator for this parametrization. I highlight the benefits, limitations, and specific considerations of this approach. To illustrate this methodological study, I rely on a toy problem consisting of recovering a clear image of a ring from a blurred and noisy version. I use it to illustrate the various tools and issues related to parametrization, which I apply within sampling and optimization inverse methods. Subsequently, I apply this approach to a geophysical problem and an astrophysical one to demonstrate its relevance in these domains. In geophysics, I apply this parametrization to a “downscaling” problem: the objective is to use a smooth image of the Earth’s mantle to derive a finer image containing small-scale details. To achieve this, I train the generative network on models created through geodynamic simulations; the network acts as an approximation of the simulation, more actionable for inverse problems. This application, performed on synthetic data, demonstrates the potential of this parametrization for future applications with real data. In astrophysics, I apply this approach to the generation of point spread functions (PSF). In astrophysical imaging, the PSF is an object, characteristic of the optical system, that defines how an image is blurred; estimating it accurately allows for efficient deblurring of an image. I thus parameterize the PSFs with a generative network, making the generator as an useful tool in inverse problems involving the PSF. For this case, the parametrization is performed on real PSF images, enabling to explore specific issues related to using real data.
—
The presentation will be done in French.